Features2D + Homography to find a known object
Table of Contents
Prev Tutorial: Feature Matching with FLANN
Next Tutorial: Detection of planar objects
Original author | Ana Huamán |
Compatibility | OpenCV >= 3.0 |
Goal
In this tutorial you will learn how to:
- Use the function cv::findHomography to find the transform between matched keypoints.
- Use the function cv::perspectiveTransform to map the points.
- Warning
- You need the OpenCV contrib modules to be able to use the SURF features (alternatives are ORB, KAZE, ... features).
Theory
Code
C++
This tutorial code's is shown lines below. You can also download it from here
#include <iostream>
#include "opencv2/core.hpp"
#ifdef HAVE_OPENCV_XFEATURES2D
#include "opencv2/calib3d.hpp"
#include "opencv2/highgui.hpp"
#include "opencv2/imgproc.hpp"
#include "opencv2/features2d.hpp"
#include "opencv2/xfeatures2d.hpp"
using namespace cv;
using namespace cv::xfeatures2d;
using std::cout;
using std::endl;
const char* keys =
"{ help h | | Print help message. }"
"{ input1 | box.png | Path to input image 1. }"
"{ input2 | box_in_scene.png | Path to input image 2. }";
int main( int argc, char* argv[] )
{
CommandLineParser parser( argc, argv, keys );
{
cout << "Could not open or find the image!\n" << endl;
parser.printMessage();
return -1;
}
//-- Step 1: Detect the keypoints using SURF Detector, compute the descriptors
int minHessian = 400;
Ptr<SURF> detector = SURF::create( minHessian );
std::vector<KeyPoint> keypoints_object, keypoints_scene;
Mat descriptors_object, descriptors_scene;
detector->detectAndCompute( img_object, noArray(), keypoints_object, descriptors_object );
detector->detectAndCompute( img_scene, noArray(), keypoints_scene, descriptors_scene );
//-- Step 2: Matching descriptor vectors with a FLANN based matcher
// Since SURF is a floating-point descriptor NORM_L2 is used
Ptr<DescriptorMatcher> matcher = DescriptorMatcher::create(DescriptorMatcher::FLANNBASED);
std::vector< std::vector<DMatch> > knn_matches;
matcher->knnMatch( descriptors_object, descriptors_scene, knn_matches, 2 );
//-- Filter matches using the Lowe's ratio test
const float ratio_thresh = 0.75f;
std::vector<DMatch> good_matches;
for (size_t i = 0; i < knn_matches.size(); i++)
{
if (knn_matches[i][0].distance < ratio_thresh * knn_matches[i][1].distance)
{
good_matches.push_back(knn_matches[i][0]);
}
}
//-- Draw matches
Mat img_matches;
drawMatches( img_object, keypoints_object, img_scene, keypoints_scene, good_matches, img_matches, Scalar::all(-1),
Scalar::all(-1), std::vector<char>(), DrawMatchesFlags::NOT_DRAW_SINGLE_POINTS );
//-- Localize the object
std::vector<Point2f> obj;
std::vector<Point2f> scene;
for( size_t i = 0; i < good_matches.size(); i++ )
{
//-- Get the keypoints from the good matches
obj.push_back( keypoints_object[ good_matches[i].queryIdx ].pt );
scene.push_back( keypoints_scene[ good_matches[i].trainIdx ].pt );
}
Mat H = findHomography( obj, scene, RANSAC );
//-- Get the corners from the image_1 ( the object to be "detected" )
std::vector<Point2f> obj_corners(4);
obj_corners[0] = Point2f(0, 0);
std::vector<Point2f> scene_corners(4);
perspectiveTransform( obj_corners, scene_corners, H);
//-- Draw lines between the corners (the mapped object in the scene - image_2 )
//-- Show detected matches
imshow("Good Matches & Object detection", img_matches );
waitKey();
return 0;
}
#else
int main()
{
std::cout << "This tutorial code needs the xfeatures2d contrib module to be run." << std::endl;
return 0;
}
#endif
int rows
the number of rows and columns or (-1, -1) when the matrix has more than 2 dimensions
Definition: mat.hpp:2137
Mat findHomography(InputArray srcPoints, InputArray dstPoints, int method=0, double ransacReprojThreshold=3, OutputArray mask=noArray(), const int maxIters=2000, const double confidence=0.995)
Finds a perspective transformation between two planes.
void perspectiveTransform(InputArray src, OutputArray dst, InputArray m)
Performs the perspective matrix transformation of vectors.
void imshow(const String &winname, InputArray mat)
Displays an image in the specified window.
void line(InputOutputArray img, Point pt1, Point pt2, const Scalar &color, int thickness=1, int lineType=LINE_8, int shift=0)
Draws a line segment connecting two points.
Definition: xfeatures2d.hpp:68
"black box" representation of the file storage associated with a file on disk.
Definition: core.hpp:106
Java
This tutorial code's is shown lines below. You can also download it from here
import java.util.ArrayList;
import java.util.List;
import org.opencv.calib3d.Calib3d;
import org.opencv.core.Core;
import org.opencv.core.CvType;
import org.opencv.core.DMatch;
import org.opencv.core.KeyPoint;
import org.opencv.core.Mat;
import org.opencv.core.MatOfByte;
import org.opencv.core.MatOfDMatch;
import org.opencv.core.MatOfKeyPoint;
import org.opencv.core.MatOfPoint2f;
import org.opencv.core.Point;
import org.opencv.core.Scalar;
import org.opencv.features2d.DescriptorMatcher;
import org.opencv.features2d.Features2d;
import org.opencv.highgui.HighGui;
import org.opencv.imgcodecs.Imgcodecs;
import org.opencv.imgproc.Imgproc;
import org.opencv.xfeatures2d.SURF;
class SURFFLANNMatchingHomography {
public void run(String[] args) {
String filenameObject = args.length > 1 ? args[0] : "../data/box.png";
String filenameScene = args.length > 1 ? args[1] : "../data/box_in_scene.png";
Mat imgObject = Imgcodecs.imread(filenameObject, Imgcodecs.IMREAD_GRAYSCALE);
Mat imgScene = Imgcodecs.imread(filenameScene, Imgcodecs.IMREAD_GRAYSCALE);
if (imgObject.empty() || imgScene.empty()) {
System.err.println("Cannot read images!");
System.exit(0);
}
//-- Step 1: Detect the keypoints using SURF Detector, compute the descriptors
double hessianThreshold = 400;
int nOctaves = 4, nOctaveLayers = 3;
boolean extended = false, upright = false;
SURF detector = SURF.create(hessianThreshold, nOctaves, nOctaveLayers, extended, upright);
MatOfKeyPoint keypointsObject = new MatOfKeyPoint(), keypointsScene = new MatOfKeyPoint();
Mat descriptorsObject = new Mat(), descriptorsScene = new Mat();
detector.detectAndCompute(imgObject, new Mat(), keypointsObject, descriptorsObject);
detector.detectAndCompute(imgScene, new Mat(), keypointsScene, descriptorsScene);
//-- Step 2: Matching descriptor vectors with a FLANN based matcher
// Since SURF is a floating-point descriptor NORM_L2 is used
DescriptorMatcher matcher = DescriptorMatcher.create(DescriptorMatcher.FLANNBASED);
List<MatOfDMatch> knnMatches = new ArrayList<>();
matcher.knnMatch(descriptorsObject, descriptorsScene, knnMatches, 2);
//-- Filter matches using the Lowe's ratio test
float ratioThresh = 0.75f;
List<DMatch> listOfGoodMatches = new ArrayList<>();
for (int i = 0; i < knnMatches.size(); i++) {
if (knnMatches.get(i).rows() > 1) {
DMatch[] matches = knnMatches.get(i).toArray();
if (matches[0].distance < ratioThresh * matches[1].distance) {
listOfGoodMatches.add(matches[0]);
}
}
}
MatOfDMatch goodMatches = new MatOfDMatch();
goodMatches.fromList(listOfGoodMatches);
//-- Draw matches
Mat imgMatches = new Mat();
Features2d.drawMatches(imgObject, keypointsObject, imgScene, keypointsScene, goodMatches, imgMatches, Scalar.all(-1),
//-- Localize the object
List<Point> obj = new ArrayList<>();
List<Point> scene = new ArrayList<>();
List<KeyPoint> listOfKeypointsObject = keypointsObject.toList();
List<KeyPoint> listOfKeypointsScene = keypointsScene.toList();
for (int i = 0; i < listOfGoodMatches.size(); i++) {
//-- Get the keypoints from the good matches
obj.add(listOfKeypointsObject.get(listOfGoodMatches.get(i).queryIdx).pt);
scene.add(listOfKeypointsScene.get(listOfGoodMatches.get(i).trainIdx).pt);
}
MatOfPoint2f objMat = new MatOfPoint2f(), sceneMat = new MatOfPoint2f();
objMat.fromList(obj);
sceneMat.fromList(scene);
double ransacReprojThreshold = 3.0;
Mat H = Calib3d.findHomography( objMat, sceneMat, Calib3d.RANSAC, ransacReprojThreshold );
//-- Get the corners from the image_1 ( the object to be "detected" )
Mat objCorners = new Mat(4, 1, CvType.CV_32FC2), sceneCorners = new Mat();
float[] objCornersData = new float[(int) (objCorners.total() * objCorners.channels())];
objCorners.get(0, 0, objCornersData);
objCornersData[0] = 0;
objCornersData[1] = 0;
objCornersData[2] = imgObject.cols();
objCornersData[3] = 0;
objCornersData[4] = imgObject.cols();
objCornersData[5] = imgObject.rows();
objCornersData[6] = 0;
objCornersData[7] = imgObject.rows();
objCorners.put(0, 0, objCornersData);
Core.perspectiveTransform(objCorners, sceneCorners, H);
float[] sceneCornersData = new float[(int) (sceneCorners.total() * sceneCorners.channels())];
sceneCorners.get(0, 0, sceneCornersData);
//-- Draw lines between the corners (the mapped object in the scene - image_2 )
Imgproc.line(imgMatches, new Point(sceneCornersData[0] + imgObject.cols(), sceneCornersData[1]),
Imgproc.line(imgMatches, new Point(sceneCornersData[2] + imgObject.cols(), sceneCornersData[3]),
Imgproc.line(imgMatches, new Point(sceneCornersData[4] + imgObject.cols(), sceneCornersData[5]),
Imgproc.line(imgMatches, new Point(sceneCornersData[6] + imgObject.cols(), sceneCornersData[7]),
//-- Show detected matches
HighGui.imshow("Good Matches & Object detection", imgMatches);
HighGui.waitKey(0);
System.exit(0);
}
}
public class SURFFLANNMatchingHomographyDemo {
public static void main(String[] args) {
// Load the native OpenCV library
System.loadLibrary(Core.NATIVE_LIBRARY_NAME);
new SURFFLANNMatchingHomography().run(args);
}
}
Python
This tutorial code's is shown lines below. You can also download it from here
from __future__ import print_function
import cv2 as cv
import numpy as np
import argparse
parser = argparse.ArgumentParser(description='Code for Feature Matching with FLANN tutorial.')
parser.add_argument('--input1', help='Path to input image 1.', default='box.png')
parser.add_argument('--input2', help='Path to input image 2.', default='box_in_scene.png')
args = parser.parse_args()
img_object = cv.imread(cv.samples.findFile(args.input1), cv.IMREAD_GRAYSCALE)
img_scene = cv.imread(cv.samples.findFile(args.input2), cv.IMREAD_GRAYSCALE)
if img_object is None or img_scene is None:
print('Could not open or find the images!')
exit(0)
#-- Step 1: Detect the keypoints using SURF Detector, compute the descriptors
minHessian = 400
detector = cv.xfeatures2d_SURF.create(hessianThreshold=minHessian)
keypoints_obj, descriptors_obj = detector.detectAndCompute(img_object, None)
keypoints_scene, descriptors_scene = detector.detectAndCompute(img_scene, None)
#-- Step 2: Matching descriptor vectors with a FLANN based matcher
# Since SURF is a floating-point descriptor NORM_L2 is used
matcher = cv.DescriptorMatcher_create(cv.DescriptorMatcher_FLANNBASED)
knn_matches = matcher.knnMatch(descriptors_obj, descriptors_scene, 2)
#-- Filter matches using the Lowe's ratio test
ratio_thresh = 0.75
good_matches = []
for m,n in knn_matches:
if m.distance < ratio_thresh * n.distance:
good_matches.append(m)
#-- Draw matches
img_matches = np.empty((max(img_object.shape[0], img_scene.shape[0]), img_object.shape[1]+img_scene.shape[1], 3), dtype=np.uint8)
cv.drawMatches(img_object, keypoints_obj, img_scene, keypoints_scene, good_matches, img_matches, flags=cv.DrawMatchesFlags_NOT_DRAW_SINGLE_POINTS)
#-- Localize the object
obj = np.empty((len(good_matches),2), dtype=np.float32)
scene = np.empty((len(good_matches),2), dtype=np.float32)
for i in range(len(good_matches)):
#-- Get the keypoints from the good matches
obj[i,0] = keypoints_obj[good_matches[i].queryIdx].pt[0]
obj[i,1] = keypoints_obj[good_matches[i].queryIdx].pt[1]
scene[i,0] = keypoints_scene[good_matches[i].trainIdx].pt[0]
scene[i,1] = keypoints_scene[good_matches[i].trainIdx].pt[1]
H, _ = cv.findHomography(obj, scene, cv.RANSAC)
#-- Get the corners from the image_1 ( the object to be "detected" )
obj_corners = np.empty((4,1,2), dtype=np.float32)
obj_corners[0,0,0] = 0
obj_corners[0,0,1] = 0
obj_corners[1,0,0] = img_object.shape[1]
obj_corners[1,0,1] = 0
obj_corners[2,0,0] = img_object.shape[1]
obj_corners[2,0,1] = img_object.shape[0]
obj_corners[3,0,0] = 0
obj_corners[3,0,1] = img_object.shape[0]
scene_corners = cv.perspectiveTransform(obj_corners, H)
#-- Draw lines between the corners (the mapped object in the scene - image_2 )
cv.line(img_matches, (int(scene_corners[0,0,0] + img_object.shape[1]), int(scene_corners[0,0,1])),\
(int(scene_corners[1,0,0] + img_object.shape[1]), int(scene_corners[1,0,1])), (0,255,0), 4)
cv.line(img_matches, (int(scene_corners[1,0,0] + img_object.shape[1]), int(scene_corners[1,0,1])),\
(int(scene_corners[2,0,0] + img_object.shape[1]), int(scene_corners[2,0,1])), (0,255,0), 4)
cv.line(img_matches, (int(scene_corners[2,0,0] + img_object.shape[1]), int(scene_corners[2,0,1])),\
(int(scene_corners[3,0,0] + img_object.shape[1]), int(scene_corners[3,0,1])), (0,255,0), 4)
cv.line(img_matches, (int(scene_corners[3,0,0] + img_object.shape[1]), int(scene_corners[3,0,1])),\
(int(scene_corners[0,0,0] + img_object.shape[1]), int(scene_corners[0,0,1])), (0,255,0), 4)
#-- Show detected matches
cv.imshow('Good Matches & Object detection', img_matches)
cv::String findFile(const cv::String &relative_path, bool required=true, bool silentMode=false)
Try to find requested data file.
void drawMatches(InputArray img1, const std::vector< KeyPoint > &keypoints1, InputArray img2, const std::vector< KeyPoint > &keypoints2, const std::vector< DMatch > &matches1to2, InputOutputArray outImg, const Scalar &matchColor=Scalar::all(-1), const Scalar &singlePointColor=Scalar::all(-1), const std::vector< char > &matchesMask=std::vector< char >(), DrawMatchesFlags flags=DrawMatchesFlags::DEFAULT)
Draws the found matches of keypoints from two images.
CV_EXPORTS_W Mat imread(const String &filename, int flags=IMREAD_COLOR)
Loads an image from a file.
Explanation
Result
- And here is the result for the detected object (highlighted in green). Note that since the homography is estimated with a RANSAC approach, detected false matches will not impact the homography calculation.
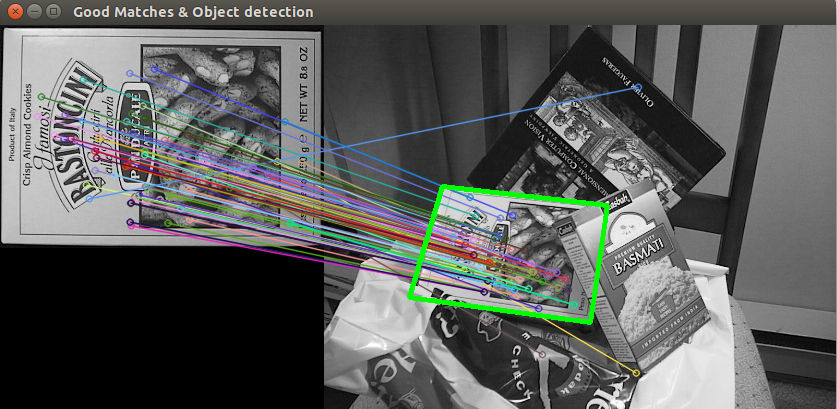